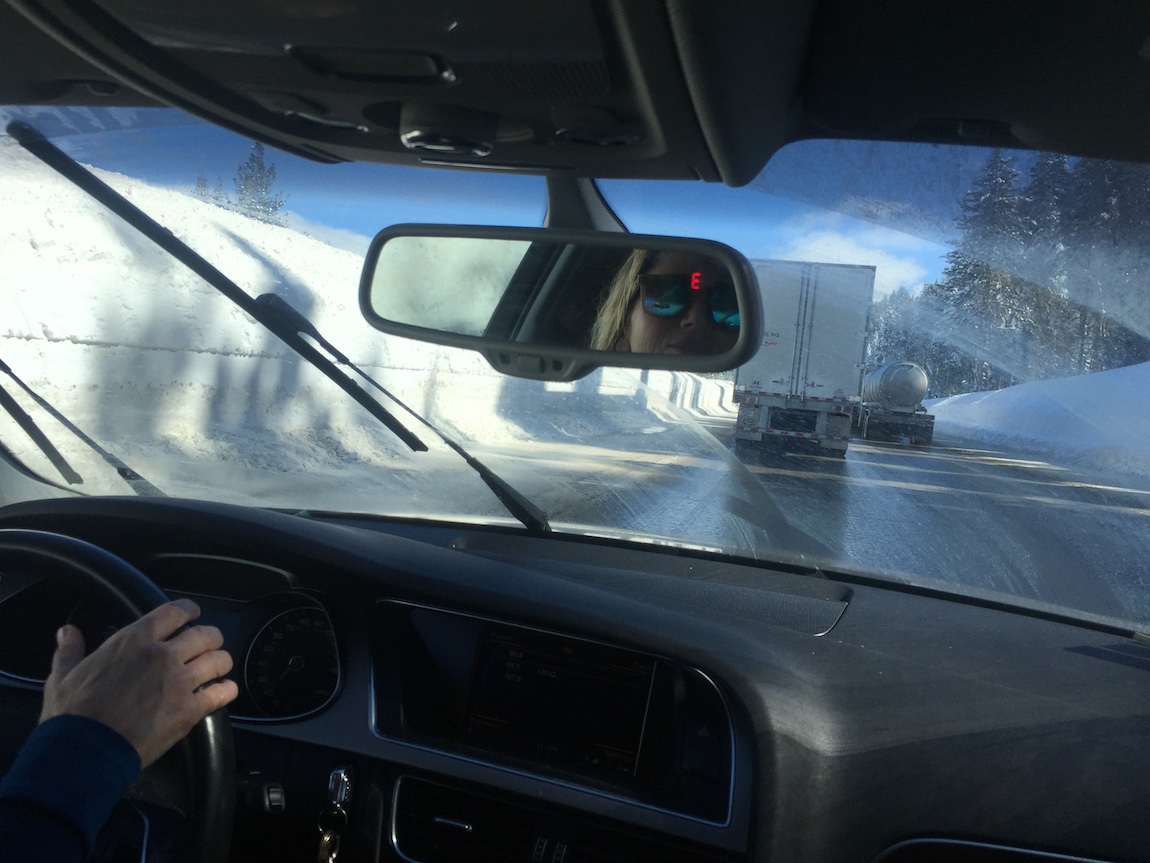
It’s the mantra of big data and machine learning. The past predicts the future. But what if the future is a total departure from the past? In that case, does machine learning become really stupid about the present? To answer this question, let’s turn to something as simple as the 200-mile drive from San Francisco to Lake Tahoe. Tech could make this drive efficient, predictable. Save time, fuel, even lives. But instead, when winter blizzards hit, it’s like a data white-out. Nobody, not even Google or Apple, can see where they’re going.
Skiers in the San Francisco Bay Area live this truth every weekend. It’s been a record-breaking season for snow in the Sierras, a skier’s dream that has also brought a slew of road closures, accidents, and chain control over the perilous Donner Summit. This is a textbook case study in all the shortcomings of tech’s much-touted last mile. For the millions who drive every year to ski in the Lake Tahoe area, the opportunity for innovation is obvious. Google Maps, Apple Maps, and all of the embedded tech they rely on, are routine liars, telling us that the road is smooth sailing when it’s clogged with chain control, fraught with accidents, or even worse, simply closed.
This happens so consistently these days that you wonder why some brainy powder hound at Google on their creative 20 percent time doesn’t do something about it. In this obvious failure of predictive analytics, the past is a totally unreliable source of data. Especially with freakish weather events caused by global warming. Consider for instance this scenario:
A wintry Sunday afternoon at Lake Tahoe, where the weekend population swells by a quarter million people. Let’s say you’re among the fifteen thousand or so ripping it at Squaw Valley, then planning to head back home to the Bay Area. When there’s no traffic and no snow, the drive takes about three hours. Google Maps says you’re good to go at 1pm. But by 2pm, the app has added 30 minutes to your trip. Tens of thousands of other skiers are clogging the road by now. You hop in the car. It starts snowing, which you could have predicted from the 70 percent chance of precipitation shown in your weather app. The California State Route 89 feeder road to Interstate 80 from Squaw begins backing up. Here comes the tipping point. Around 2:30pm, Caltrans decides to enforce chain control near Donner Summit. Now, you are inching along on CA-89. At 3:00, Google has added an hour to your trip estimate. Only an hour? you wonder, as you inch forward, for the next half hour. By 3:30pm, you know it’s all bogus. The drive from Squaw to the summit, just 20 miles, takes an excruciating three hours. The algorithm failed to predict or accurately gauge real-time events – such as an entirely predictable snow storm, chain control, and the normal Sunday afternoon crunch of cars on the road. At an average speed of a little over 20 mph, it takes you nine hours to drive home – three times Google’s original projection, and several hours beyond your threshold.
There’s a colossal opportunity in this failure. For starters, the Caltrans website doesn’t appear to have been updated since the 1990s, and only gives the most rudimentary one- to two-sentence notices on weather or closures, with gaps between updates ranging between 12 and 24 hours. That’s an absurdly deficient service, given the importance to industry and commerce of keeping I-80 open. Imagine instead pulling up your TahoeTrippin™ app, a sophisticated UX based on statistical analysis, with inputs such as weather, accidents, day of the week, number of visitors already in Tahoe, etc, and variable components on the user end (desired time of departure, arrival, etc). TahoeTrippin™ would offer two or three probabilities for your experience, because you can’t truly anticipate the weather or accidents.
What if you could enter in projected departure times. Run virtual experiments. As in, checking the estimated drive time if you leave at 1pm, 2pm, or 4pm? This could be displayed in a color-coded, design thinking style. Instead of relying on Caltrans, TahoeTrippin™ would tap into local, real-time government, commercial, and crowdsourced inputs for road closures, weather, and traffic problems.
The current approaches aren’t up to the challenge. Major storms are lined up for the next two weeks. The weather and drive to the Sierras is bound to get even weirder. It’s time to get started on TahoeTrippin™. Who wants to help build it?